Keynotes
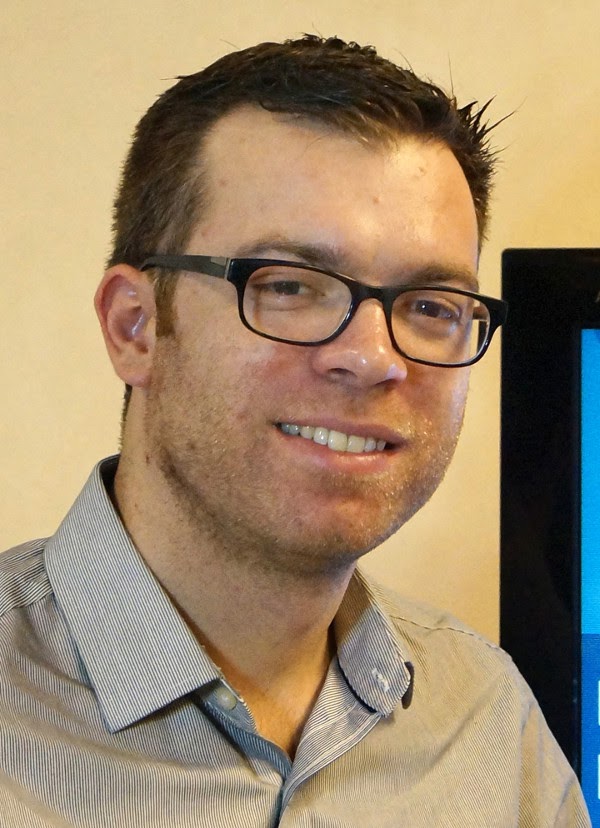
Damien Querlioz, Research Director at CNRS, Adjunct Professor at Université Paris-Saclay
In-Memory Computing: A Path to Energy-Efficient and Trustworthy Embedded AI
Abstract: Artificial intelligence (AI) holds tremendous promise for edge applications, but its high energy demands impede adoption in areas such as medical implants and advanced brain–machine interfaces. Traditional computing systems are especially inefficient for AI workloads due to the significant energy cost of transferring data between memory and processing units. In this talk, we explore how in-memory and near-memory computing can overcome these challenges, enabling low-power, embedded AI. We focus on the tight integration of logic and memory to drastically reduce energy consumption, highlighting emerging non-volatile memory technologies, such as memristors, magnetic memory, and phase-change memory, that have reached a level of maturity sufficient for fully functional in-memory computing systems. These devices pave the way for robust, low-power digital neural networks while also allowing analog in-memory computing, in which neural network operations are performed inherently through fundamental electrical laws. Although these technologies introduce variability, we show how Bayesian methods can mitigate—and sometimes even leverage—these imperfections. We also discuss recent progress in local learning algorithms, including Equilibrium Propagation, which promise efficient on-chip learning using in-memory computing. Finally, we review current challenges and outline future directions for adopting these technologies at the architectural level to achieve trustworthy, energy-efficient AI.
Biography: Damien Querlioz is a CNRS Research Director at the Centre de Nanosciences et de Nanotechnologies of Université Paris-Saclay and CNRS. His research focuses on novel usages of emerging non-volatile memory and other nanodevices, in particular relying on inspirations from biology and machine learning. He received his predoctoral education at Ecole Normale Supérieure, Paris and his PhD from Université Paris-Sud in 2009. Before his appointment at CNRS, he was a Postdoctoral Scholar at Stanford University and at the Commissariat à l’Energie Atomique.
Damien Querlioz is the coordinator of the interdisciplinary INTEGNANO research group, with colleagues working on all aspects of nanodevice physics and technology, from materials to systems. In 2016, he was the recipient of an ERC Starting Grant to develop the concept of natively intelligent memory. In 2017, he received the CNRS Bronze medal. He has also been a co-recipient of the 2017 IEEE Guillemin-Cauer Best Paper Award and of the 2018 IEEE Biomedical Circuits and Systems Best Paper Award.
Prof. Jan Rabaey, University of California, Berkeley
The “Compute Continuum” and its Energetics
Abstract: Over the past decades, we have seen computing evolve from an isolated and centralized endeavor to an environment that exploits the heterogeneity of geographically distributed resources to dynamically execute parallel and distributed applications. The heterogeneity spans from massively parallel compute centers executing complex generative AI models to tiny wirelessly-connected sensors embedded in robotics, self-driving cars, the environment and the human body. In a sense, the world around us is being transformed into an all-embracing computing network that provides intelligent assessment, helps manage resources and operates robotic entities, all while intimately keeping the human in the loop (the “compute continuum”). This evolution is rapidly and exponentially accelerating at all levels of the compute hierarchy. Yet that growth is most likely to be stunted by energy limitations. Already today the demand for computational power exceeds availability as well as the capability of delivering that power to the right place and time. In this presentation, we explore how technology innovation can help to address some of these concerns with different solutions for the high-performance platforms and the distributed sensory systems. In the end though, it is only a system-level perspective – considering the compute continuum as a connected dynamic distributed and evolutionary system – that will deliver a sustainable long-term solution.
Biography: Jan Rabaey is a Professor in the Graduate School in the EECS Department the University of California at Berkeley, after being the holder of the Donald O. Pederson Distinguished Professorship at the same institute for over 30 years. He is a founding director of the Berkeley Wireless Research Center (BWRC) and the Berkeley Ubiquitous SwarmLab, and has served as the Electrical Engineering Division Chair at Berkeley twice. In 2019, he also became the CTO of the System-Technology Co-Optimization (STCO) Division of IMEC, Belgium.
Prof. Rabaey has made high-impact contributions to a number of fields, including low power integrated circuits, advanced wireless systems, mobile devices, sensor networks, and ubiquitous computing. Some of the systems he helped envision include the infoPad (a forerunner of the iPad), PicoNets and PicoRadios (IoT avant-la-lettre), the Swarm (IoT on steroids), Brain-Machine interfaces and the Human Intranet. His current interests include the conception of the next-generation distributed systems, as well as the exploration of the interaction between the cyber and the biological worlds.
He is the primary author of the influential “Digital Integrated Circuits: A Design Perspective” textbook that has served to educate hundreds of thousands of students all over the world. He is the recipient of numerous awards among which the 2009 EDAA lifetime achievement award, is a Life Fellow of the IEEE, and has been involved in a broad variety of start-up ventures.
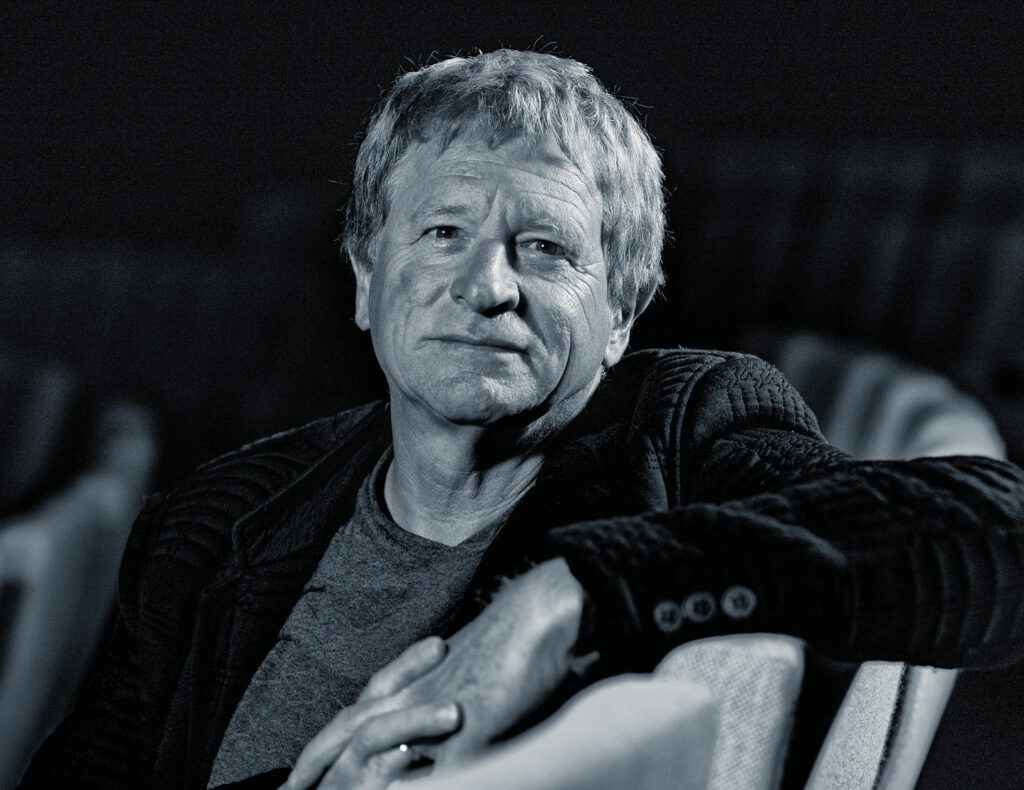
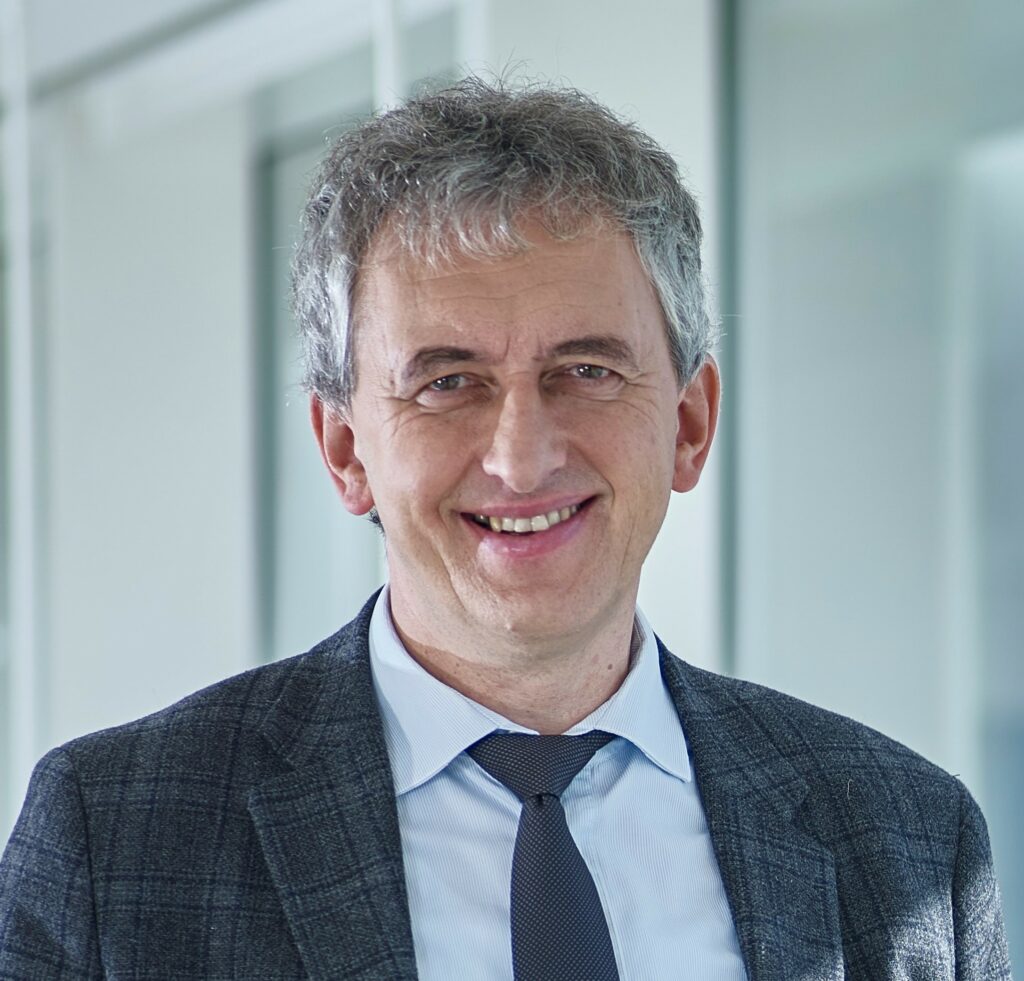
Prof. Georges Gielen, KU Leuven, Belgium
Designing analog integrated circuits: inspiration in nature or synthesized by AI?
Abstract: In this digitizing world, analog/mixed-signal integrated circuits remain key in all applications where electronics meet the physical world. While the performance requirements keep rising, however, analog circuit design remains difficult, time consuming and error-prone, and these circuits don’t scale well to (sub-)nanometer CMOS. So how can we bridge this gap and how will future analog circuits be designed? This presentation will describe progress along two different tracks. One where inspiration from human nature is used to create low-area low-energy circuits. Examples are bio-inspired solutions for sensing readout, such as in biomedical applications for e-skin or neuromodulation. The other track uses (generative) AI and machine learning to create and optimize analog circuits automatically. But is this hype or feasible? Will we still need analog designers in the future?
Biography: Georges G.E. Gielen received the MSc and PhD degrees in Electrical Engineering from the Katholieke Universiteit Leuven (KU Leuven), Belgium, in 1986 and 1990, respectively. Currently, he is Full Professor in the MICAS research division at the Department of Electrical Engineering (ESAT) at KU Leuven. From August 2013 until July 2017 he served as Vice‐Rector for the Group of Sciences, Engineering and Technology. In 2018 he was visiting professor at UC Berkeley and Stanford University. From 2020 to 2024 he served as Chair of the Department of Electrical Engineering (ESAT) at KU Leuven. His research interests are in the design of analog and mixed‐signal integrated circuits, and especially in analog and mixed‐signal CAD tools and design automation, including modeling, simulation, optimization and synthesis as well as testing.
He is a frequently invited speaker/lecturer and coordinator/partner of several (industrial) research projects in this area, including an ERC Advanced Grant. He has (co‐)authored 10 books and more than 700 publications in edited books, international journals and conference proceedings. He is a 1997 Laureate of the Belgian Royal Academy of Sciences, Literature and Arts in the discipline of Engineering. He is Fellow of the IEEE since 2002, and received the IEEE CAS Mac Van Valkenburg award in 2015 and the IEEE CAS Charles Desoer award in 2020, as well as the EDAA Achievement Award in 2021. He is an elected member of the Royal Flemish Academy of Belgium in the class of Technical Sciences, and of the Academia Europaea.